AI Model Reconstructs 3D Human Faces from DNA with High Accuracy
A new study has introduced Difface, an AI-based framework capable of reconstructing 3D human facial images directly from DNA, demonstrating high fidelity in linking genetic variants to phenotypic facial features. Developed by researchers in China and published in Advanced Science, the model combines contrastive multimodal learning, transformer and spiral convolution architectures, and a generative diffusion process to map single nucleotide polymorphisms (SNPs) to realistic facial geometries.
Model Architecture and Validation
Difface was trained on 9,674 paired SNP profiles and 3D facial scans from Han Chinese individuals. It maps both genetic data and facial surfaces into a shared low-dimensional feature space using contrastive learning. The generative component then reconstructs a 3D face from this embedding using a diffusion model. Incorporating auxiliary variables such as age, sex, and BMI further improved reconstruction accuracy.
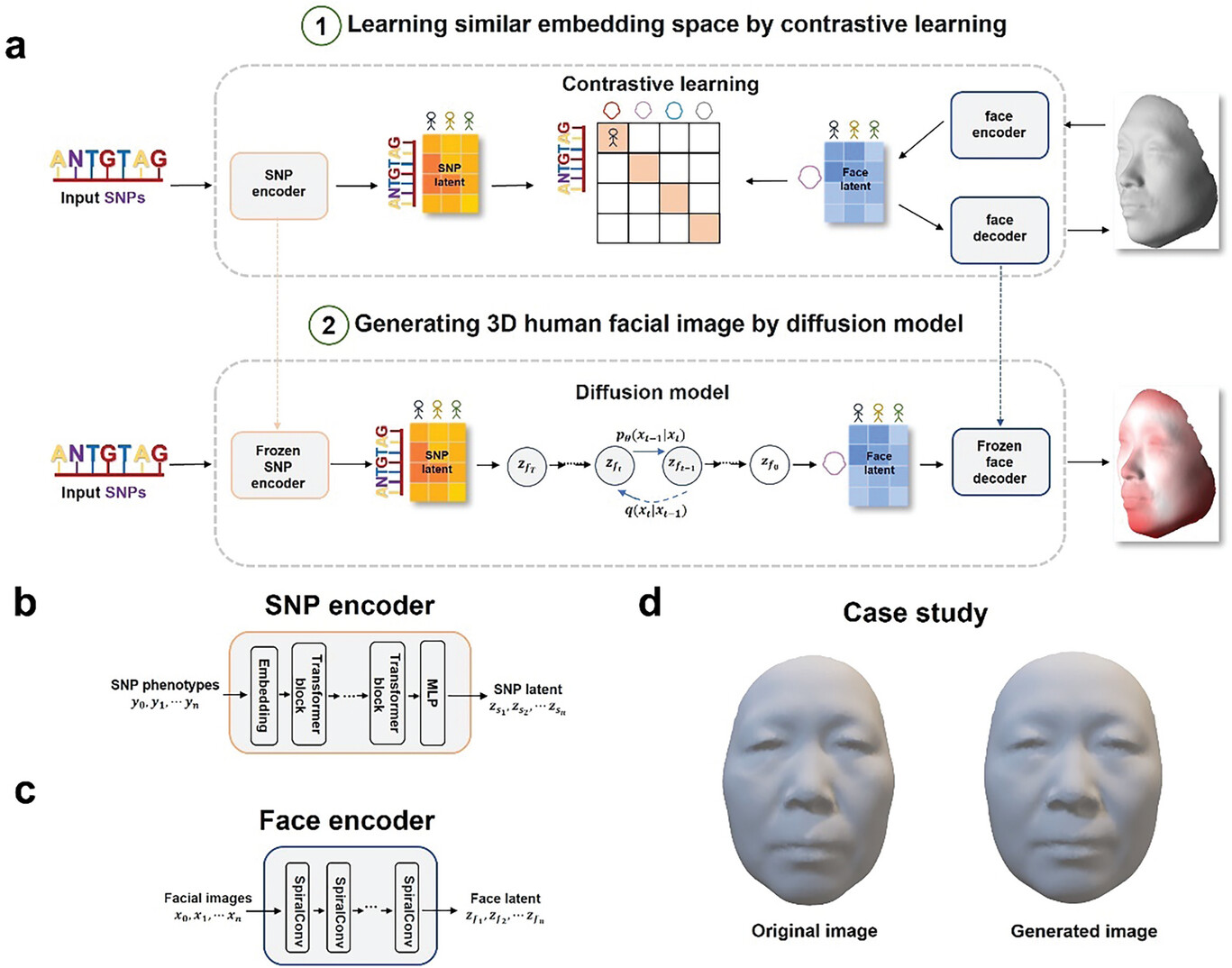
Overview of the Difface framework, which aligns SNP and facial data in a shared feature space using contrastive learning, then generates 3D facial reconstructions from DNA via a diffusion model and fixed decoders.
Benchmarking against prior models, Difface achieved a rank-1 identification rate of 3.33% and an AUC of 80.7% in verification tasks, outperforming previous approaches including those by Mahdi et al. and Sero et al. Facial reconstruction error (mean Euclidean distance) was 3.52 mm using SNPs alone, decreasing to 2.93 mm when including phenotypic covariates. The model also accurately captured facial changes across age groups and achieved high classification accuracy in distinguishing facial features.
Limitations
Difface maintained high facial reconstruction fidelity even when trained on partial SNP inputs, though accuracy declined below 70% SNP completeness. The model also achieved near-parity in facial diversity compared to real-world datasets, with a DPP diversity score of 0.996 versus 0.9999 for actual images.
Importantly, Difface also demonstrated interpretability. Using SHAP values and GWAS enrichment analysis, researchers identified specific SNPs strongly linked to traits such as nasal shape and cheekbone structure. These features were biologically validated and showed alignment with known developmental pathways in gene ontology terms.
Applications and Future Scope
Difface offers potential applications in forensic science, particularly for individual identification based on degraded or partial genetic samples, and in personalized medicine by providing insights into genetic contributions to craniofacial structure. A blinded study found that volunteers could correctly match synthetic and real faces with up to 75.6% accuracy in five-choice lineups.
Although developed on a genetically homogeneous East Asian population, the model's architecture is designed to be generalizable. The authors note that training on multi-ethnic datasets will be critical for expanding applicability and ensuring fairness in downstream use cases.
Ethical Considerations
Despite its technical achievements, Difface highlights profound concerns around genomic privacy, data re-identification, and biometric surveillance. The ability to reconstruct faces from de-identified genomic data raises new risks of misuse in legal, healthcare, and insurance settings. The authors call for clear ethical guidelines and cross-sectoral dialogue to address these implications.
Topics: AI & Digital