Harvard and Roche Develop Foundation AI Model to Predict and Explain Immunotherapy Response Across 33 Cancer Types
Researchers from Harvard Medical School and Roche have developed COMPASS, a foundation AI model designed to predict patient response to immune checkpoint inhibitors (ICIs) across 33 cancer types and six ICI therapies. The model addresses a long-standing challenge in oncology of identifying which patients will benefit from immunotherapy by learning biological concepts directly from tumor transcriptomes and linking them to treatment outcomes.
Trained on over 10,000 pre-treatment tumor RNA-seq samples and fine-tuned on 16 clinical cohorts, COMPASS outperformed 22 baseline methods and surpassed commonly used biomarkers such as PD-L1 expression and tumor mutational burden (TMB). In a phase II urothelial cancer trial, it achieved a hazard ratio of 4.7 (p < 0.0001) for survival prediction, compared to 1.7 and 1.5 for TMB and PD-L1, respectively.
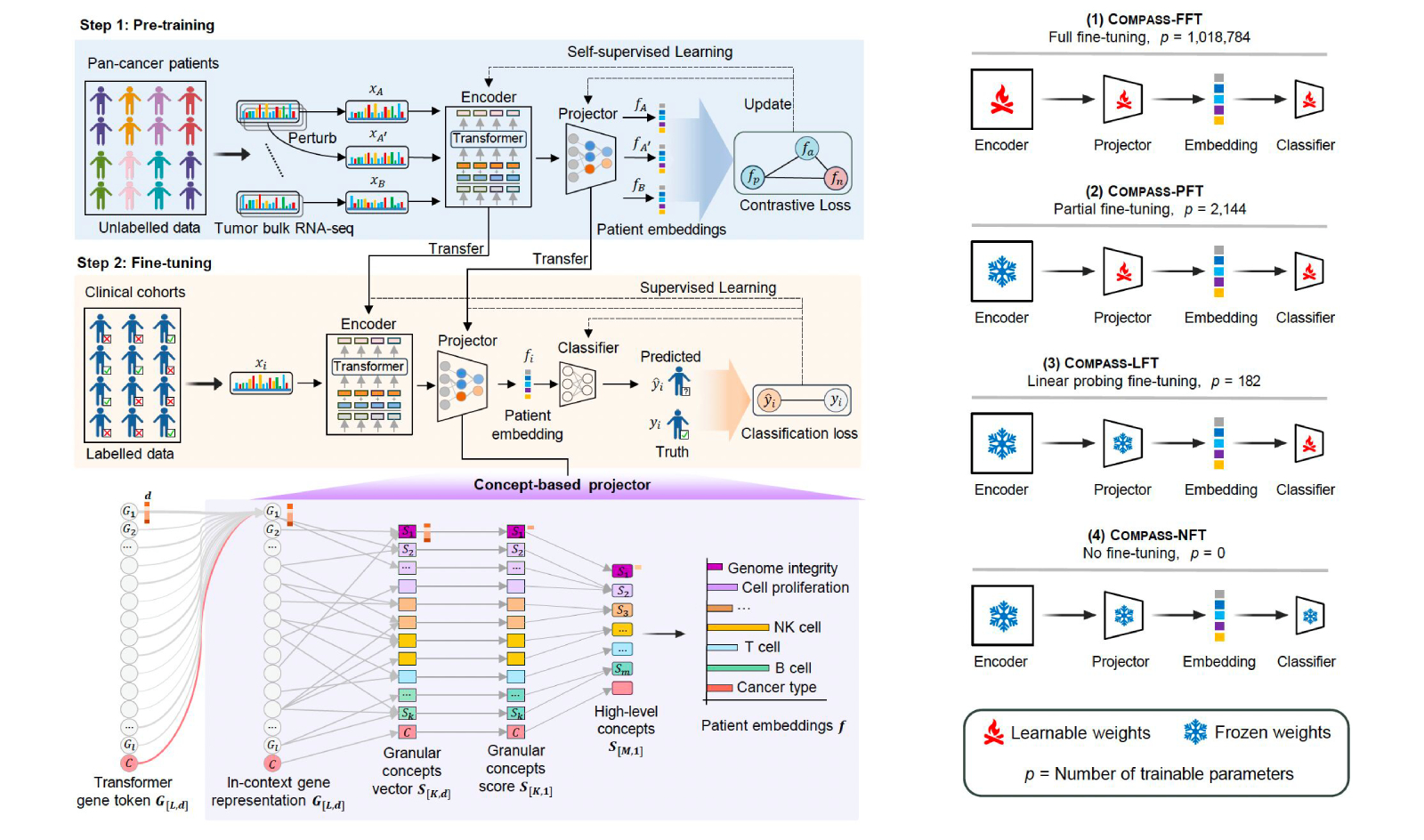
Figure 1: Architecture of the COMPASS model for interpretable immunotherapy response prediction. Gene expression data is encoded into biologically grounded tumor-immune (TIME) concepts via a hierarchical projector, enabling classification of responders versus non-responders with flexible fine-tuning options for clinical adaptation.
The model is designed for clinical generalization and interpretability, integrating transfer learning with a concept bottleneck approach that maps gene expression to biologically meaningful latent variables. This enables not only improved prediction across unseen cancer types and therapies, but also insight into why patients respond or fail. For example, COMPASS uncovered distinct resistance mechanisms in immune-inflamed non-responders—including TGF-β signaling, vascular exclusion, CD4+ T cell dysfunction, and B cell deficiency.
COMPASS was evaluated using leave-one-cohort-out and cross-cohort transfer benchmarks, showing high accuracy and robustness even in small and heterogeneous datasets. Its predictions remained consistent across diverse cancer types, biopsy sites, and sequencing pipelines, supporting its potential as a pan-cancer clinical tool for immunotherapy stratification.
The model’s release marks a step toward more clinically aligned, biologically grounded AI systems in oncology, with implications for drug development, patient selection, and precision immunotherapy. An interactive tool for personalized response explanation is also publicly available for researchers.
Topics: AI & Digital